Abductive task abstractions in physical problem-solving
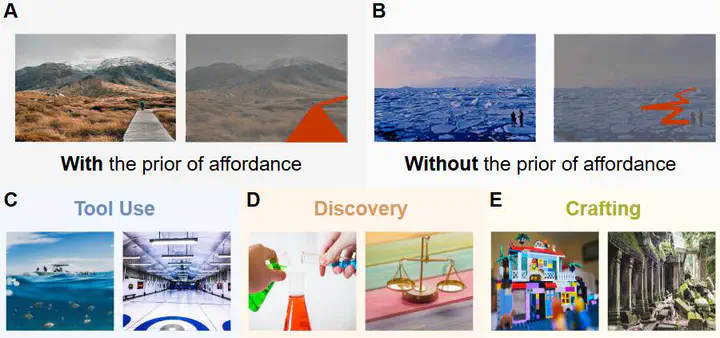
Humans solve problems with ease for two primary reasons. For one, we can infer problem context by leveraging object-level prior (henceforth prior for brevity), such as semantics, affordance, and physics. For another, we can simplify the problem context to a task-oriented abstract representation (henceforth task abstraction for brevity), such as only considering the routine graph instead of the entire city map when traveling by subway.
Unfortunately, priors are latent or inaccessible in many real-world problems, thus difficult to separate them from task abstraction. Here we introduce an experimental procedure to probe how people generate task abstraction without priors.
We devise a hierarchical generative model that accounts for human behavior of task abstraction from the first principles. We design experiments that require participants to solve different problems instructed with a goal (Exp1) or goal and constraint (Exp2) to testify model hypothesis. Model fitting on data of Exp1 significantly shows that task abstractions converge on the same family of goals regardless of variants, yet the policies for solving are diverse.
Thus, we further show that the diversity of policies may come from personal preference, independent of the generation of task abstraction, echoing the hierarchy of our model. In Exp2, variants of constraint generally do not transform task abstraction, defending the generality of our findings.
With the model hypothesis checked and the comparison with alternate accounts, we suggest that with the absence of priors, people may construct task abstraction from common sense on tasks in a top-down manner. Our findings may lead to general discussions on "knowing how to solve an unknown problem before starting to solve it".
MetaQ and PPO are chosen to be taught from scratch in the ProbSol environment. The MetaQ agent fails to complete all tasks across all trajectories, but the PPO agent succeeds in the two tasks. The success rate indicates that the reinforcement learning agent is incapable of learning or producing the appropriate strategy to complete the task. It does not learn anything but rather remembers the answer based on the gradient. Thus, we conclude that the gradient-learning-based RL cannot generate task abstraction for the ProbSol environment, let alone produce viable strategies.
Besides hierarchical modeling, we employ imitation learning to study the properties of task abstraction, to maintain the generality of our analysis. We find that the behavior of BC agent is to some extent consistent with that of human subjects. We view the activation vector of the penultimate hidden layer as the feature of task abstraction extracted by the BC agent. In the same task, features of different policies cannot be separated from each other. These observations by imitation learning echo our findings by the hierarchical modeling.